Node2Vec 图嵌入: 《悲惨世界》人物关系
参考资料:
Elior Cohen 代码:https://github.com/eliorc/node2vec
Elior Cohen 博客:https://maelfabien.github.io/machinelearning/graph_5/
1. 环境准备
安装工具包
1
| !pip install node2vec networkx numpy matplotlib
|
导入工具包
1 2 3 4 5 6 7 8 9 10 11 12
| import networkx as nx
import numpy as np
import random
import matplotlib.pyplot as plt
|
2. 数据
初始化
1 2 3 4 5
|
G = nx.les_miserables_graph()
|
NodeView(('Napoleon', 'Myriel', 'MlleBaptistine', 'MmeMagloire', 'CountessDeLo', 'Geborand', 'Champtercier', 'Cravatte', 'Count', 'OldMan', 'Valjean', 'Labarre', 'Marguerite', 'MmeDeR', 'Isabeau', 'Gervais', 'Listolier', 'Tholomyes', 'Fameuil', 'Blacheville', 'Favourite', 'Dahlia', 'Zephine', 'Fantine', 'MmeThenardier', 'Thenardier', 'Cosette', 'Javert', 'Fauchelevent', 'Bamatabois', 'Perpetue', 'Simplice', 'Scaufflaire', 'Woman1', 'Judge', 'Champmathieu', 'Brevet', 'Chenildieu', 'Cochepaille', 'Pontmercy', 'Boulatruelle', 'Eponine', 'Anzelma', 'Woman2', 'MotherInnocent', 'Gribier', 'MmeBurgon', 'Jondrette', 'Gavroche', 'Gillenormand', 'Magnon', 'MlleGillenormand', 'MmePontmercy', 'MlleVaubois', 'LtGillenormand', 'Marius', 'BaronessT', 'Mabeuf', 'Enjolras', 'Combeferre', 'Prouvaire', 'Feuilly', 'Courfeyrac', 'Bahorel', 'Bossuet', 'Joly', 'Grantaire', 'MotherPlutarch', 'Gueulemer', 'Babet', 'Claquesous', 'Montparnasse', 'Toussaint', 'Child1', 'Child2', 'Brujon', 'MmeHucheloup'))
77
可视化
1 2 3 4
| plt.figure(figsize=(15,14)) pos = nx.spring_layout(G, seed=5) nx.draw(G, pos, with_labels=True) plt.show()
|
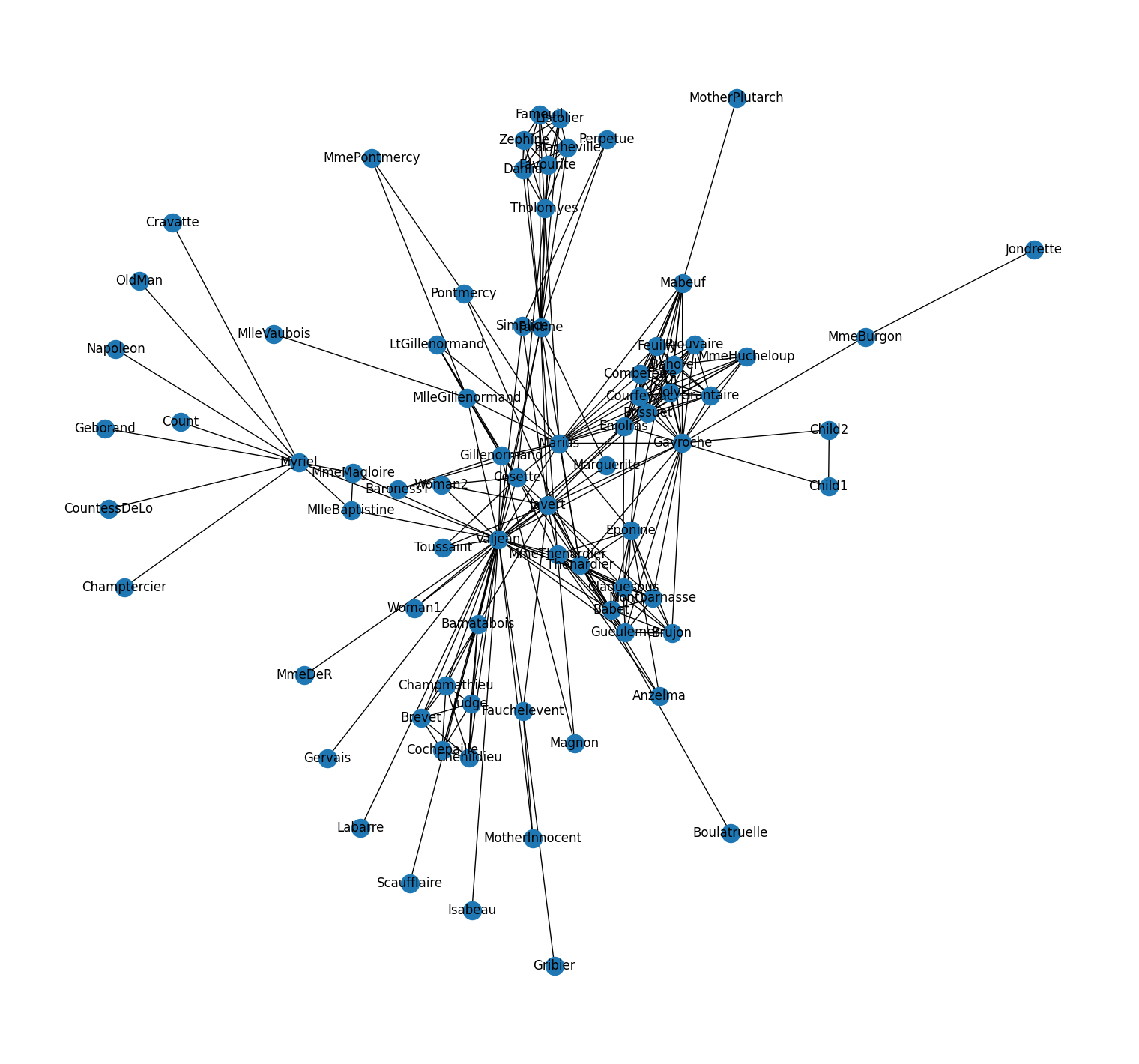
3. 构建 Node2Vec 模型
Node2Vec 嵌入
1 2 3 4 5 6 7 8 9 10 11 12 13 14 15 16 17 18 19 20 21 22 23
| from node2vec import Node2Vec
n2v = Node2Vec(G, dimensions=32, p=2, q=0.5, walk_length=30, num_walks=600, workers=4 )
model = n2v.fit(window=3, min_count=1, batch_words=4 )
X = model.wv.vectors
|
Computing transition probabilities: 100%|█████████████████████████████████████████████| 77/77 [00:00<00:00, 4949.22it/s]
Generating walks (CPU: 1): 100%|██████████| 150/150 [00:00<00:00, 330.07it/s]
Generating walks (CPU: 4): 100%|██████████| 150/150 [00:00<00:00, 337.63it/s]
Generating walks (CPU: 2): 100%|██████████| 150/150 [00:00<00:00, 326.68it/s]
Generating walks (CPU: 3): 100%|██████████| 150/150 [00:00<00:00, 328.33it/s]
(77, 32)
KMeans 聚类可视化
Kmeans 聚类算法讲解:https://www.bilibili.com/video/BV1HJ411P7cs/
1 2 3 4 5 6 7 8 9 10 11 12
|
from sklearn.cluster import KMeans import numpy as np
cluster_labels = KMeans(n_clusters=3).fit(X).labels_ print(cluster_labels)
|
[2 1 1 1 2 2 1 1 1 2 2 2 1 2 1 1 1 2 2 2 1 2 2 2 2 2 2 2 2 2 2 2 1 1 0 0 2
1 2 0 0 0 0 2 1 2 1 1 1 2 1 2 2 2 2 2 2 1 1 2 2 2 1 2 2 2 1 2 2 2 1 2 2 2
2 2 2]
1 2 3 4 5 6 7 8 9 10 11
| colors = [] nodes = list(G.nodes)
for node in nodes: idx = model.wv.key_to_index[str(node)] colors.append(cluster_labels[idx]) plt.figure(figsize=(15,14)) pos = nx.spring_layout(G, seed=10) nx.draw(G, pos, node_color=colors, with_labels=True) plt.show()
|
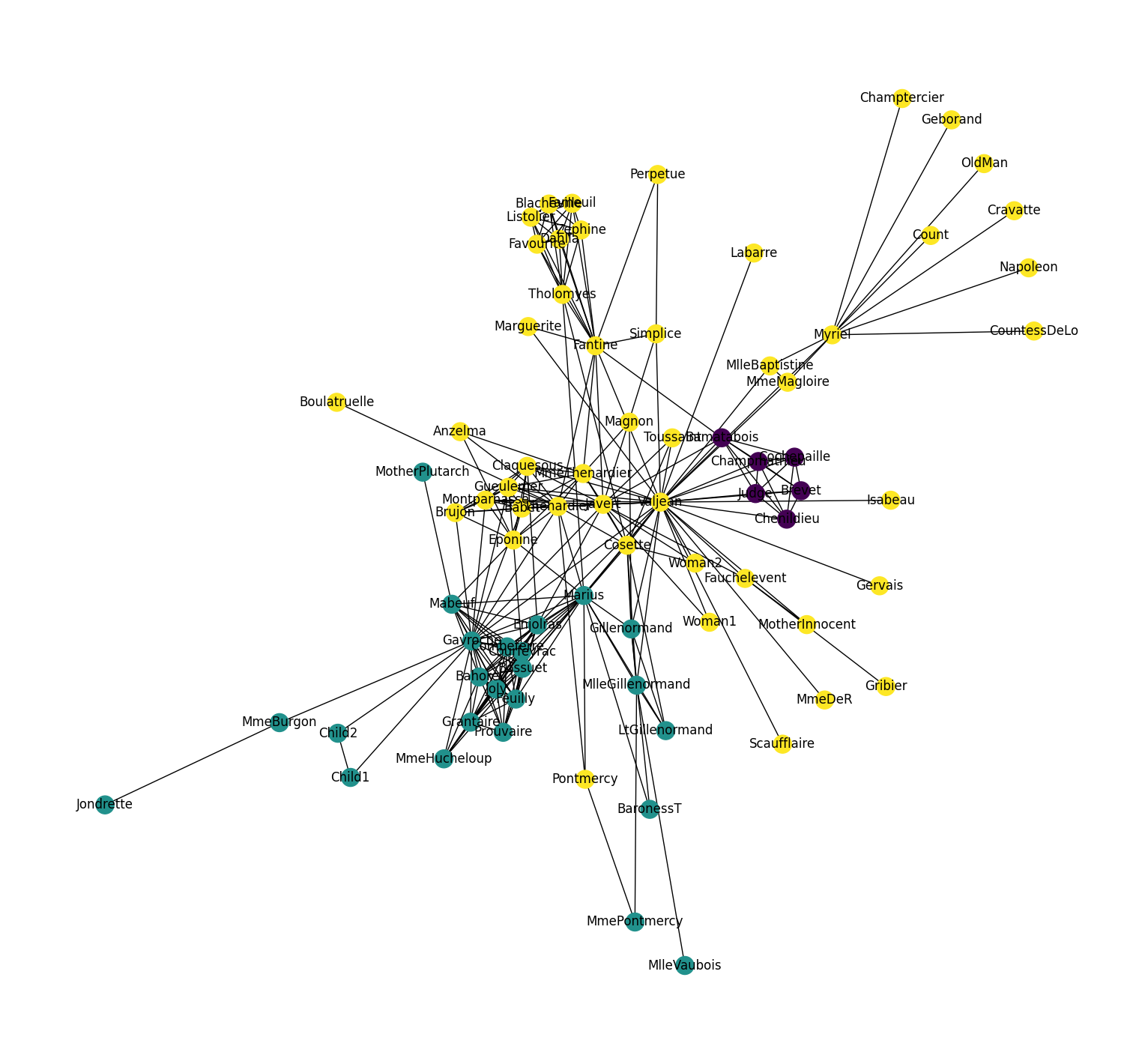
PCA 降维可视化
1 2 3 4 5 6 7 8 9 10 11 12 13
| from sklearn.decomposition import PCA pca = PCA(n_components=2) embed_2d = pca.fit_transform(X)
plt.figure(figsize=(6,5)) plt.scatter(embed_2d[:,0], embed_2d[:,1]) plt.show()
|
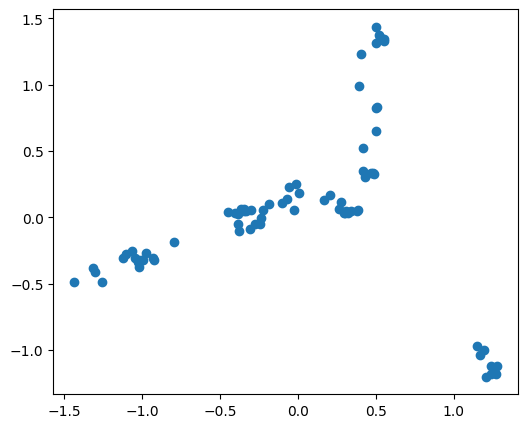
分析 Embedding
1
| model.wv.get_vector('Napoleon').shape
|
(32,)
1 2
| model.wv.get_vector('Napoleon')
|
array([ 0.16437183, -0.05719004, 0.7591357 , 0.5262734 , -0.28540358,
0.3609386 , -0.0202415 , 0.59487563, 0.16668592, -0.36358935,
-0.31636822, -0.24678008, 0.07872051, 0.2453149 , 0.47021243,
0.14235401, -0.24516489, -0.06233275, -0.7360416 , 0.5266324 ,
0.25197074, 0.8208004 , 0.1812625 , 0.4639347 , -0.02985335,
0.46994781, -0.01152692, 0.50284547, 0.06440508, -0.58088064,
0.41836476, 0.20175534], dtype=float32)
1 2
| model.wv.most_similar('Napoleon')
|
[('Count', 0.9734092950820923),
('Geborand', 0.9711518883705139),
('Champtercier', 0.9699333310127258),
('CountessDeLo', 0.9690977931022644),
('OldMan', 0.9581858515739441),
('Cravatte', 0.9463319182395935),
('MmeMagloire', 0.9301953911781311),
('MlleBaptistine', 0.9220814108848572),
('Myriel', 0.8496583104133606),
('Labarre', 0.43950924277305603)]
1
| model.wv.similar_by_word('Napoleon')
|
[('Count', 0.9734092950820923),
('Geborand', 0.9711518883705139),
('Champtercier', 0.9699333310127258),
('CountessDeLo', 0.9690977931022644),
('OldMan', 0.9581858515739441),
('Cravatte', 0.9463319182395935),
('MmeMagloire', 0.9301953911781311),
('MlleBaptistine', 0.9220814108848572),
('Myriel', 0.8496583104133606),
('Labarre', 0.43950924277305603)]
1 2
| model.wv.similarity('Napoleon', 'Napoleon')
|
1.0
1
| model.wv.similarity('Napoleon', 'OldMan')
|
0.9581857
4. 对连接做 Embedding
1 2 3 4 5 6 7
| from node2vec.edges import HadamardEmbedder
edges_embs = HadamardEmbedder(keyed_vectors=model.wv)
edges_embs[('Napoleon', 'OldMan')]
|
array([ 0.02212512, 0.01081607, 0.49410883, 0.25259754, 0.09594341,
0.08860359, 0.00081227, 0.37484962, 0.03543219, 0.09974428,
0.06573805, 0.01136922, 0.00543256, 0.04992007, 0.1747883 ,
-0.00262563, 0.01901744, 0.01046612, 0.63943666, 0.2961468 ,
0.11473472, 0.5845541 , 0.05060175, 0.23471911, 0.00586544,
0.29180774, -0.00227471, 0.16711667, -0.00148958, 0.39783025,
0.15422934, 0.0504636 ], dtype=float32)
1 2
| edges_kv = edges_embs.as_keyed_vectors()
|
Generating edge features: 100%|███████████████████████████████████████████████| 3003/3003.0 [00:00<00:00, 248123.53it/s]
1
| len(edges_kv.index_to_key)
|
3003
["('Valjean', 'Valjean')",
"('Marius', 'Valjean')",
"('Enjolras', 'Valjean')",
"('Courfeyrac', 'Valjean')",
...]
1 2
| edges_kv.most_similar(str(('Napoleon', 'OldMan')))
|
[("('Count', 'OldMan')", 0.9915876388549805),
("('CountessDeLo', 'OldMan')", 0.9897369742393494),
("('CountessDeLo', 'Napoleon')", 0.9895244240760803),
("('Geborand', 'Napoleon')", 0.9881743788719177),
("('Champtercier', 'OldMan')", 0.9877184629440308),
("('Geborand', 'OldMan')", 0.9872523546218872),
("('Count', 'Napoleon')", 0.9845482707023621),
("('OldMan', 'OldMan')", 0.9842210412025452),
("('Napoleon', 'Napoleon')", 0.9839144349098206),
("('Cravatte', 'OldMan')", 0.9834887385368347)]